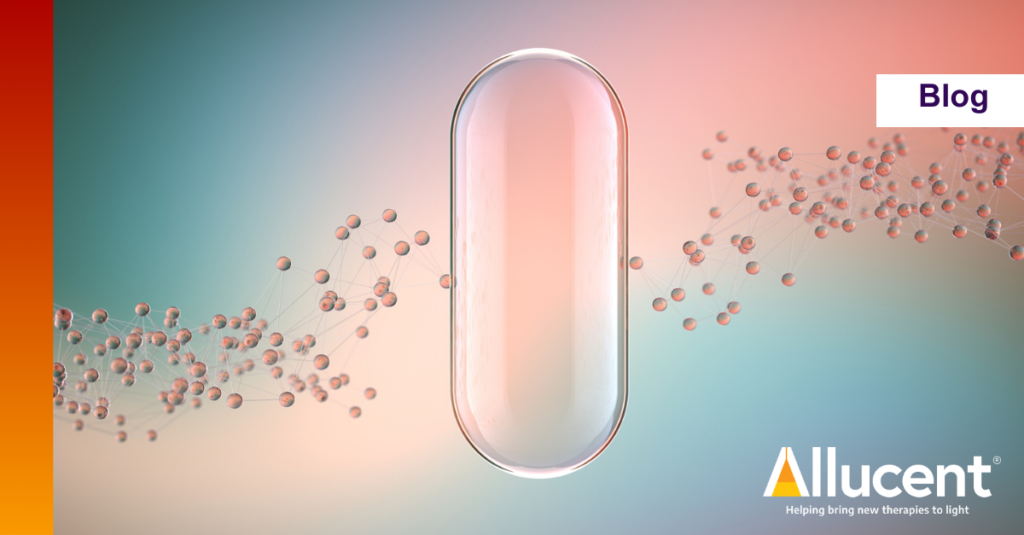
By Neha Bhise (Associate Director, Clinical Pharmacology), Soha Freidy (Pharmacometrician I), and Jessica Roberts (Director, Pharmacometrics)
Mechanistic pharmacokinetic (PK)/pharmacodynamic (PD) modeling is a quantitative approach that has been successfully used to identify potential for success across various stages of drug discovery and development. These models are useful to distinguish characteristics of promising compounds from those associated with development risks. Mechanistic PK/PD modeling provides a quantitative relationship between PK and therapeutic effect of the drug by mathematically describing the biological mechanism of action (MOA) and relevant target biology. Mechanistic PK/PD models quantify the causal pathways connecting drug concentrations and therapeutic effect (Danhof et.al. 2008). These pathways include various essential components such as target site distribution, target binding and activation, PD interactions, transduction, and homeostatic feedback mechanisms. In addition, mechanistic PK/PD modeling can also characterize the interaction of drug effect with disease progression.
Mechanistic PK/PD models recognize that either PK or receptor binding and/or physiological levels of receptors control drug action (efficacy or safety). The quantitative relationship between PK/PD enhances the model predictive capabilities that can significantly impact drug development by designing more targeted and effective therapies (Daryaee et.al. 2019, Grant et.al. 2023). Based on the stage of drug development and the intended application, the parameters for developing the models can be estimated/obtained from either nonclinical data (in vitro and/or animal), literature data, or clinical data.
Since mechanistic PK/PD models account for biological mechanisms, they distinguish themselves from empirical models in that they account for drug-specific parameters and physiological/biological parameters. This gives mechanistic PK/PD models improved properties for extrapolation and prediction versus empirical PK/PD models, which leads to more confident decision-making, especially during discovery and early development.
Why Is Mechanistic PK/PD Modeling Important?
While PK modeling quantitatively describes the process of drug’s absorption and disposition in the body, PD modeling describes the time course of the drug’s pharmacological effects, considering the drug’s MOA and the biology of the body. PK/PD modeling can help in predicting, in a quantitative manner, the relationship of the drug exposure and its response and help characterize the influences of drug-specific, delivery-specific, and physiological and pathological system-specific parameters on this relationship. While these PK/PD models or population PK models are data driven models, mechanistic PK/PD models, even in the absence of extensive data (especially at the early stages of development), could provide valuable information at the early stages of development by leveraging the information regarding the target biology, or the available data from the literature, if any. Mechanistic PK/PD modeling, which considers the pathophysiological mechanisms behind the PK/PD relationships, enables the evaluation of various properties of the delivery systems (e.g., extended-release formulations, liposomal drugs, antibody drug conjugates, modified proteins) on the in vivo drug effect by allowing the separation of the drug-specific and system-specific parameters. This helps provide competitive advantage during the drug development process because it allows the generation and testing of hypotheses much more quickly, offering valuable insights in the early stages of drug discovery and development.
Applications of Mechanistic PK/PD Modeling During Drug Development
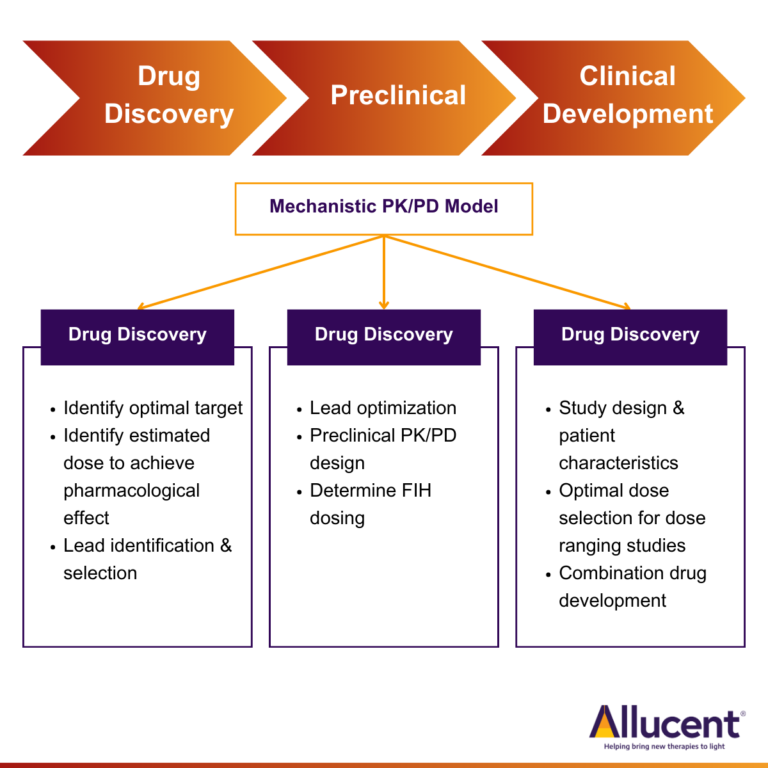
Mechanistic PK/PD modeling can help across various stages of drug discovery and development.
Target Identification and Lead Candidate Selection
Using mechanistic modeling in lieu of multiple in vitro and in vivo studies to help select the right target and optimal lead candidate can result in considerable time and cost saving. Mechanistic modeling can be used to assess therapeutic concept feasibility of a target based on its expression levels, turnover, and site of expression among others. Additionally, key optimal drug specific properties like binding affinity, in vitro potency, clearance, and distribution to site of action can be identified using mechanistic modeling to help select a lead candidate for further assessment in preclinical animal models. For example, Chimalakonda et. al. used a mechanistic PK/PD model to identify key target and drug related factors that can impact duration of target inhibition. They were able to show that improvement in target binding affinity reaches point of diminishing returns and further improvement in affinity does not alter the magnitude and duration of target inhibition (Chimalakonda et.al. 2013) The learnings from this theoretical assessment can be used to identify a lead candidate that will result in optimal inhibition of the target. These learnings can also provide critical insights regarding the doses that need to be evaluated in preclinical animal studies to enable adequate characterization of an exposure-response relationship. Since PK/PD data from these animal studies is often used to predict human efficacious doses and define the safety multiples, designing preclinical efficacy studies that help identify optimal efficacious dose and dosing regimen is important to assess the likelihood of success of the investigational agent.
Translational Modeling to Support Human Dose Projections
Mechanistic models built using data from nonclinical animal models can be “translated” to simulate the effects in humans by replacing key biological parameters (i.e., target expression, turnover) and drug specific properties (i.e., binding affinity, clearance) with those observed in humans and thus can aid in determining first-in-human (FIH) dose. As clinical data becomes available, these models can be further refined and updated and support decisions regarding patient selection and doses that need to be evaluated in proof-of-concept clinical studies . For example, translational pharmacokinetic and tumor growth inhibition model of pembrolizumab was developed based on mouse data and human physiological data and then used to predict the efficacious dose range for pembrolizumab (Lindauer et.al. 2017).
Mechanistic PK/PD modeling can also help with competitive differentiation by identifying the advantages of the new molecule compared to competitor molecules, e.g., dosing advantage or better therapeutic index. By specifically comparing the predicted optimal binding affinity, target coverage and off-target effects of the new molecule to that of the marketed biologics, these models can help define the criteria for development. A mechanistic PK/PD model developed for candidate selection for anti-CCL20 antibody was used to simulate a range of drug properties and dosing regimens and to support competitive differentiation (Kapitanov et.al. 2021).
Optimizing Formulation and Accelerated Clinical Development
Mechanistic PK/PD modeling supported the clinical development of a 3-month extended-release formulation of paliperidone palmitate (PP3M) (Samtani et.al. 2016 ). Drawing from insights gathered in the Phase I study of PP3M, a comprehensive population PK model was developed. Through mechanistic PK/PD modeling and simulations, researchers identified a dose that closely mirrored the PK profile of the 1-month formulation (PP1M) observed in the Phase III study. This strategy rooted in PK matching postulated that drug effects hinged on plasma drug concentration surpassing a specific target concentration, a concept supported by prior PP1M PK/PD investigations. Intriguingly, the PK/PD analysis of PP1M and a limited dataset from the single-dose Phase I of PP3M used to predict dose in Phase III, rendering a Phase II dose-finding study for PP3M unnecessary. The Phase III study successfully achieved the predicted PK and efficacy outcomes, underscoring the triumph of mechanistic PK/PD modeling in expediting the clinical development of novel drug delivery systems and concomitantly curtailing costs.
Thus, the incorporation of mechanistic PK/PD modeling at every stage of drug development not only accelerates hypothesis testing but also provides researchers with a competitive edge in designing more effective and safer therapies.
Allucent’s Use of Mechanistic PK/PD Modeling
Mechanistic PK/PD modeling has potential to address multiple questions during early drug development that can impact the predicted efficacious dose, the optimal target, and the optimal drug property. Since these key components are critical for success of the drug development program, mechanistic PK/PD modeling plays a critical role in optimizing and accelerating the process of drug development.
Allucent’s team of experienced clinical and quantitative translational pharmacologists and pharmacometricians are experts in modeling and simulation, including mechanistic PK/PD modeling.
For additional content on pharmacokinetics and pharmacodynamics, check out these other blogs:
- Pharmacometrics (PMx), Pharmacokinetics, Pharmacodynamics (PK/PD)
- What is Population Pharmacokinetic (popPK) Analysis?
References
- Mechanism-based pharmacokinetic-pharmacodynamic modeling: biophase distribution, receptor theory, and dynamical systems analysis. Meindert Danhof, Joost de Jongh, Elizabeth C M De Lange, Oscar Della Pasqua, Bart A Ploeger, Rob A Voskuy.
- Pharmacokinetic-pharmacodynamic models that incorporate drug-target binding kinetics. Fereidoon Daryaee, Peter J Tonge.
- Mechanistic PK/PD modeling to address early-stage biotherapeutic dosing feasibility questions. Joshuaine Grant, Fei Hua, Joshua F Apgar, John M Burke, Diana H Marcantonio.
- Factors Influencing Magnitude and Duration of Target Inhibition Following Antibody Therapy: Implications in Drug Discovery and Development. Anjaneya P. Chimalakonda, Rajbharan Yadav and Punit Marathe.
- Translational Pharmacokinetic/Pharmacodynamic Modeling of Tumor Growth Inhibition Supports Dose-Range Selection of the Anti–PD-1 Antibody Pembrolizumab. A Lindauer, CR Valiathan, K Mehta, V Sriram, R de Greef, J Elassaiss-Schaap and DP de Alwis.
- A Mechanistic Site-Of-Action Model: A Tool for Informing Right Target, Right Compound, And Right Dose for Therapeutic Antagonistic Antibody Programs. Georgi I Kapitanov, Jeffrey R Chabot, Jatin Narula, Mahua Roy, Hendrik Neubert, Joe Palandra, Vahid Farrokhi, Jay S Johnson, Robert Webster, Hannah M Jones.
- Prospective dose selection and acceleration of paliperidone palmitate 3-month formulation development using a pharmacometric bridging strategy. Mahesh N Samtani, Partha Nandy, Paulien Ravenstijn, Bart Remmerie, An Vermeulen, Alberto Russu, Peter D’hoore, Ellen Z Baum, Adam Savitz, Srihari Gopal, David Hough.
Additional Reading
- The role of mechanism-based pharmacokinetic-pharmacodynamic (PK-PD) modelling in translational research of biologics. Balaji M. Agoram, Steven W. Martin and Piet H. van der Graaf.
- Pharmacokinetic/Pharmacodynamic Modeling for Drug Development in Oncology. Elena Garralda, MD, Rodrigo Dienstmann, MD, and Josep Tabernero, MD, PhD.
- Diversity of Mechanism-Based Pharmacodynamic Models. Donald E. Mager, Elzbieta Wyska and William J. Jusko.
- Characteristics of indirect pharmacodynamic models and applications to clinical drug responses. Amarnath Sharma and William J. Jusko.